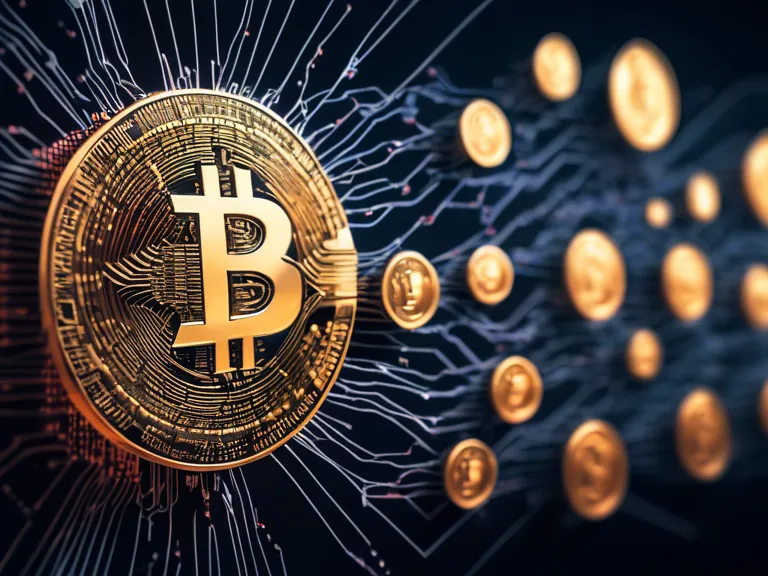
Introduction
Cryptocurrency forecasting using machine learning has gained significant popularity in recent years as traders and investors seek to leverage advanced technologies to predict market movements and make informed decisions. By utilizing historical data, statistical models, and machine learning algorithms, it is possible to generate forecasts that can help users optimize their trading strategies and maximize profits.
Importance of Cryptocurrency Forecasting
Cryptocurrency markets are known for their volatility, making them a lucrative yet risky investment. By incorporating machine learning techniques into forecasting models, traders can gain insights into market trends, price movements, and potential risks. This can help them make more informed decisions and reduce the impact of emotional bias on their trading activities.
Data Collection and Preparation
The first step in cryptocurrency forecasting using machine learning is to collect relevant data from various sources such as exchanges, social media platforms, news outlets, and technical indicators. This data may include historical price data, trading volumes, market sentiment, and other relevant factors. Once the data is collected, it needs to be cleaned, preprocessed, and structured for analysis.
Feature Selection and Engineering
Feature selection plays a crucial role in building accurate forecasting models. Relevant features such as price trends, trading volumes, market capitalization, and sentiment analysis can be extracted from the data and used as inputs for machine learning algorithms. Feature engineering techniques such as normalization, scaling, and transformation may also be applied to enhance the predictive power of the models.
Machine Learning Algorithms
Various machine learning algorithms can be employed for cryptocurrency forecasting, including linear regression, support vector machines, decision trees, random forests, and neural networks. Each algorithm has its strengths and weaknesses, and the choice of algorithm depends on the complexity of the problem and the characteristics of the data. Ensemble methods and deep learning techniques can also be used to improve forecasting accuracy.
Model Training and Evaluation
Once the features are selected and the algorithms are chosen, the next step is to train the model on historical data and evaluate its performance. This involves splitting the data into training and testing sets, tuning hyperparameters, and measuring the model's accuracy, precision, recall, and other metrics. Cross-validation techniques can be used to validate the model and prevent overfitting.
Forecasting and Decision Making
After the model is trained and evaluated, it can be used to generate forecasts for future cryptocurrency prices and trends. Traders and investors can leverage these forecasts to make informed decisions on buying, selling, or holding assets. It is important to continuously monitor the model's performance and update it with new data to ensure its relevance and accuracy.
Conclusion
Cryptocurrency forecasting using machine learning offers a powerful tool for traders and investors to navigate the volatile cryptocurrency markets. By leveraging historical data, advanced algorithms, and predictive modeling techniques, users can gain valuable insights into market trends and make data-driven decisions. While no forecasting model is perfect, incorporating machine learning can significantly enhance trading strategies and improve overall performance in the cryptocurrency market.