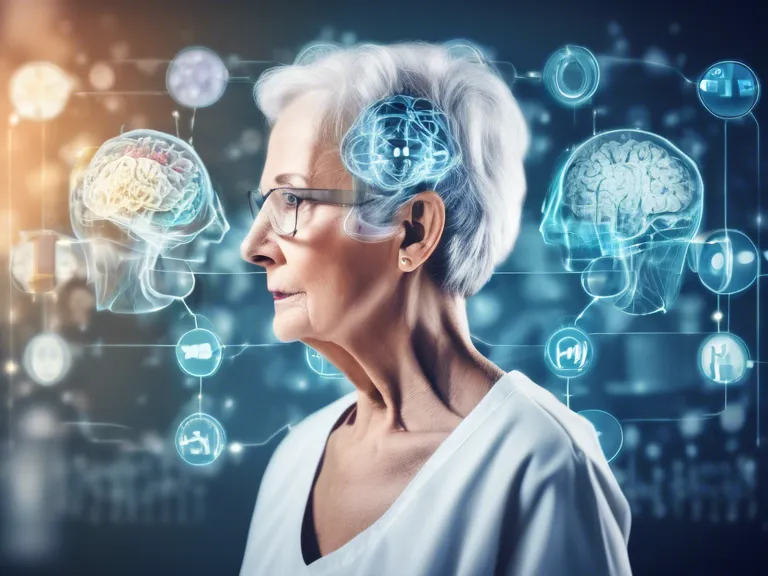
Introduction
In recent years, the field of machine learning has made significant advancements in predicting health risks in aging populations. With the increasing prevalence of chronic diseases and the growing aging population worldwide, there is a pressing need for accurate and timely predictions to improve healthcare outcomes and reduce healthcare costs. Machine learning algorithms have shown great promise in analyzing large datasets to identify patterns and trends that can help predict health risks in aging populations. This article explores the applications of machine learning in predicting health risks in aging populations and the potential impact on healthcare.
Understanding Health Risks in Aging Populations
As individuals age, they become more susceptible to various health risks and chronic conditions such as cardiovascular diseases, diabetes, and cognitive decline. Identifying these risks early on can help healthcare providers intervene and provide appropriate care to improve health outcomes and quality of life. Traditional methods of risk assessment often rely on subjective evaluations and retrospective data analysis, which may not be sufficient for accurate predictions in aging populations. Machine learning offers a data-driven approach that can leverage large datasets to identify patterns and relationships that may not be apparent through traditional methods.
Applications of Machine Learning in Predicting Health Risks
Machine learning algorithms can be applied to various types of healthcare data, including electronic health records, medical imaging, genetic data, and wearable devices. By analyzing these diverse datasets, machine learning models can identify predictive biomarkers, risk factors, and disease progression patterns in aging populations. For example, predictive models can analyze a combination of clinical data, lifestyle factors, and genetic information to assess an individual's risk of developing a specific condition over time. These models can help healthcare providers tailor interventions and treatment plans to each individual's unique health profile, leading to more personalized and effective care.
Challenges and Opportunities
While machine learning shows great potential in predicting health risks in aging populations, there are several challenges that need to be addressed. One of the main challenges is the interpretability of machine learning models, as complex algorithms may produce predictions that are difficult to explain to healthcare providers and patients. Additionally, issues related to data privacy, security, and bias in algorithmic predictions need to be carefully considered to ensure ethical and responsible use of machine learning in healthcare.
Despite these challenges, the opportunities presented by machine learning in predicting health risks in aging populations are vast. By harnessing the power of big data and advanced algorithms, healthcare providers can gain valuable insights into population health trends, disease progression, and treatment outcomes. This information can inform preventive strategies, early interventions, and personalized care plans for aging individuals, ultimately leading to improved health outcomes and reduced healthcare costs.
Conclusion
In conclusion, machine learning has the potential to revolutionize the prediction of health risks in aging populations by enabling accurate, data-driven insights that can guide healthcare decision-making. By leveraging machine learning algorithms to analyze diverse healthcare datasets, healthcare providers can identify predictive patterns and risk factors that may not be apparent through traditional methods. While there are challenges to overcome, the opportunities for improving healthcare outcomes in aging populations are immense. As machine learning continues to evolve and mature, it holds great promise for transforming the way we predict and manage health risks in aging populations.