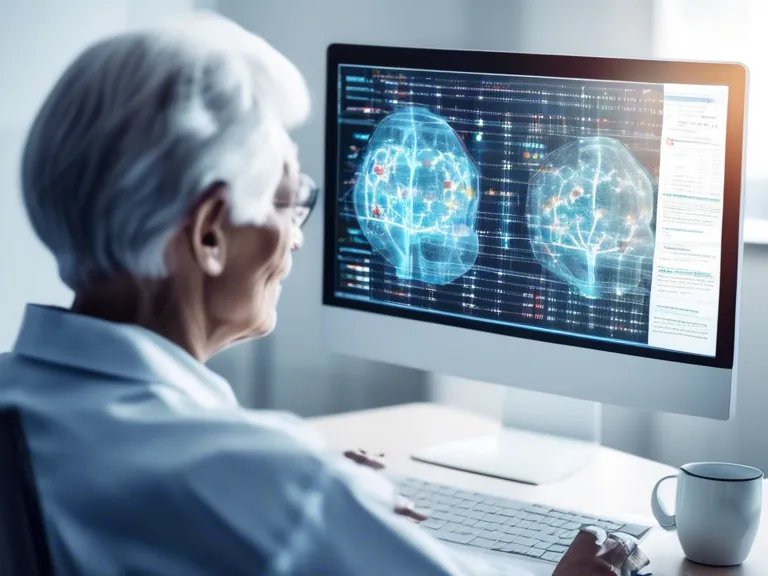
Introduction
Machine learning has revolutionized the way we approach various industries, including healthcare. In aged care domains, where predicting health risks is crucial for providing timely interventions and personalized care, machine learning algorithms play a significant role. By analyzing vast amounts of data, machine learning models can identify patterns and trends that may indicate potential health risks in elderly individuals. This article explores the applications of machine learning in predicting health risks in aged domains and the benefits it offers to both healthcare providers and patients.
Understanding Health Risks in Aged Domains
As individuals age, they are more susceptible to a range of health issues such as chronic diseases, cognitive decline, and mobility limitations. Early detection of these health risks can lead to better management and improved outcomes for elderly individuals. Machine learning algorithms can analyze various types of data, including medical records, sensor data, and lifestyle information, to identify factors that contribute to health risks in aged populations.
Data Collection and Preprocessing
One of the key steps in leveraging machine learning for predicting health risks in aged domains is data collection and preprocessing. Healthcare providers can gather data from electronic health records, wearable devices, and other sources to create a comprehensive dataset. Preprocessing techniques such as data cleaning, normalization, and feature engineering are essential to ensure the quality and relevance of the data for training machine learning models.
Machine Learning Models for Health Risk Prediction
Several machine learning algorithms can be used to predict health risks in aged domains, including logistic regression, support vector machines, random forests, and deep learning models. These algorithms can analyze complex relationships within the data and generate predictions about the likelihood of developing specific health conditions or experiencing adverse health outcomes. By training these models on historical data, healthcare providers can develop predictive models that can assist in identifying individuals at high risk.
Benefits of Machine Learning in Aged Care
The integration of machine learning in predicting health risks in aged domains offers several benefits. Healthcare providers can leverage predictive models to prioritize interventions, allocate resources efficiently, and deliver personalized care to elderly individuals. By identifying health risks at an early stage, healthcare professionals can implement preventive measures and interventions that can potentially improve the quality of life and reduce healthcare costs associated with managing chronic conditions.
Challenges and Considerations
While machine learning holds immense potential in predicting health risks in aged domains, there are challenges that need to be addressed. These include data privacy concerns, model interpretability, and the need for continuous model validation and updating. Healthcare providers must also consider ethical implications and ensure that the use of machine learning technologies complies with regulatory standards and best practices in healthcare.
Conclusion
Machine learning has the potential to transform the way health risks are predicted and managed in aged care domains. By harnessing the power of data and advanced algorithms, healthcare providers can enhance their ability to identify individuals at risk and provide targeted interventions that improve health outcomes for elderly populations. As technology continues to evolve, the integration of machine learning in aged care is expected to play a crucial role in shaping the future of healthcare delivery and improving the well-being of aging individuals.
american-boffin.com
bfbchamp.com
democraticcoma.com
tigrepelvar.com
charpoles.com
derbywheelblazers.com
fansfocus.net
guildnow.com
hediyeteyze.com
isprimecdn.com
kiira-korpi.net
manutd24.com
mediumtylerhenry.com
mishanghai.org
savethreestrikes.com
smilesbydavis.com
10puntos.net
band-shirt.com
icelandtrails.com
paulmarioday.com
thefunnynanny.com
bebovino.com
car-trackers.com
colbykeller.com
companyregistrationuk.com
dayoftears.com
entamee.com
kuttikattil.com
litjunkies.com
ljshen.com
nostalgic-radio.com
pcgameshackcenter.com
rachelboggia.com
vanessagritton.com
veraandrose.com
vernissage-zermatt.com
almagnon.com
angelicmedium.com
ascensoya.com
ascrenewables.com
bar-flat.com
beerandgluesticks.com
disorderedtimes.com
dokodepot.com
epochpr.com
essaylancers.com
gravure-movie.com
hawkeyespacover.com
hgwlab.com
itinerecoop.com
jeremyendoart.com
kananroadband.com
loguecorporate.com
nishikawaguchi-dosukebeworld.com
pikkupunapippuri.com
publishmyblog.com
rebius-kawai.com
salinggrove.com
sean-santiago.com
sitesbydawn.com
styledbyrida.com
thegolfcoastonline.com
thisismymilwaukee.com
breadboardmaniac.com
takasuapp.com
Anostomodo
Bonet Scissors
Cora Viral
Dippity Do Dog Mobile
Group Tie
Newberry County History
Oslo Velo
Planned and Present
Wilshire Language
CFL Magazine
Corner Kitchen
Glass of Win
Hydeout at the Wharf
Lotus Relocation
My Dee Dee's
Platinum Avtomaty Wulkan
Pride Outside
Rollout Reviews
Smile to Africa Adventure
ABC Trcker
Aloha Botts and Tots
America Noticias
Are You Kenilworthy
Ask My Condo
Charm Shiki
El Akhbaar
Health Cursor
Home Again Creative
Mi Punto PE
Patt and Billy
Rustique Online
SS Pro HK
Steamworks Espresso
Targeted Fat Loss Training
Team Hamano
Used Cars Buying
Dave Tries Ballet
Buon Grande
Criacao Sites
Perry Perkins Books
Writing Essay in AU
Ka Soku
Blood is Blood Movie
Eleanor Writes Things
The Happy Prince Beirut
Town of Witless Bay
Online Igrovoi Club
Trigeminal Neuralgia - Ronald Brisman MD
Chocolate City Burlesque
Advanced Electric Scooters
W Tougei
Aljouf Now
Arrister
Bruce Holmes Construction
Burlington Bridal
Chocolate Creative Design
Culture of Life Store
Elizabeth Cryan Photography
Fun Fresh Ideas
Kotoyuujin
Lyudia
Nakakirei
Peer AGS
Maimun88
Sinsei PV
Think Tank West
Wimax Gogo
Asperger Way
Book Rack WA
Hume Coover Studio
Pico Compressor
PPTAA
Artina Films
Bash Booths
Bicher Cancer Institute
Brickyard Theatre
Coffee with Jeff
Essential Libertarianism
Find the Light Within
Font Plus Tips
GB Product News
Homer's Auto Services
Luxellence Center
Matome Hannou
Nepal Smoking Pipe
OEM Korea Brand
Shoe Saxo
The Stock Market Blueprint
Untapped Inc.
Andres Carizza
Ashland Aerial
Brown About Town
REH Shows
Shotover Country
Toni Halonen
Trainers P
gayahidupsehat.org
Bulging Disc Fixed
Cadogan Cosmetics
D Chavannes
Kilo Pages
Loring Military Heritage Center
Modular Homes MA Planning Center
The Wheel Herb Farm
Bxtches Be Blogging
CCTV Installers London
HiFi Noise
iGo Inter
Payroll Services UK
Shop Moraga First
The Highline Restaurant
Visval Bags
BR Fittings
HD Fit Pro
Regalos a Peru
Shot Felt Round the World
BG Receptite
Photography Travel
Desperate Endeavors Movie
JetEx Charter
Amenity Papa
iSoftol
Pagar Besi
Oakfield BnB
Delight Worthy
Mantis Plant Protetion
Unryuuji
Wamsted On Energy
Doctor McAwesome
WHDMS
Jual Aged Domain
Gimena Macri
Rancho de Caldera
Vietnam Tours Lotus
A Little Bit OCD
Kingston Single Malt Society
CEI Spokane
Research Enterprises
Escape the Stall
Nippon Touhan Club
Pro Point Dies
Basement Metal
Boeren Boedel
BW Mariposa Inn
Growing Up Online
Heartland Baptist Church
Kandee Chihuahuas
Lubov Kirillovka
Lucky Dog Grooming and Boutique
Nihon Megane
Osiris The Series
Polychromatic Game
Soccer Planet JWT
Suzy Wimbourne Photography
The Hollywood Garage
Travel Alchemy