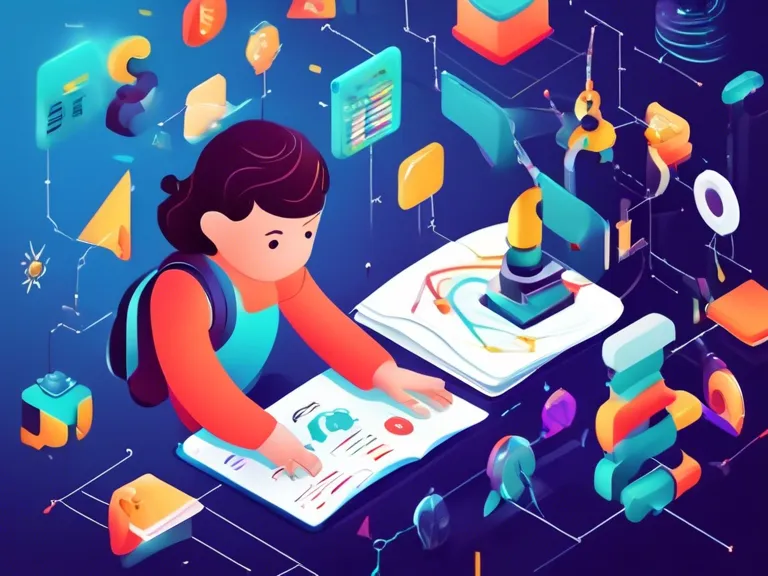
Introduction
Machine learning, a subset of artificial intelligence, has revolutionized various industries, and education is no exception. By leveraging machine learning algorithms and techniques, educators and institutions can enhance learning experiences for students, personalize learning paths, and improve overall educational outcomes.
Personalized Learning
One of the key applications of machine learning in education is personalized learning. Machine learning algorithms can analyze student data, including learning styles, preferences, strengths, and weaknesses, to create personalized learning paths for individual students. This tailored approach helps students learn at their own pace and focus on areas where they need more support, ultimately improving their understanding and retention of the material.
Adaptive Learning Platforms
Adaptive learning platforms powered by machine learning algorithms are gaining popularity in educational settings. These platforms adapt to each student's progress and performance, providing targeted feedback, additional resources, and challenges based on the student's individual needs. By continuously analyzing data and adjusting the learning experience in real-time, adaptive learning platforms help students stay engaged and motivated while maximizing their learning outcomes.
Intelligent Tutoring Systems
Machine learning has enabled the development of intelligent tutoring systems that simulate one-on-one personalized instruction. These systems use algorithms to assess student responses, identify misconceptions, and provide immediate feedback and guidance. By emulating the benefits of human tutoring, intelligent tutoring systems can help students master difficult concepts more effectively and efficiently.
Predictive Analytics
Predictive analytics, powered by machine learning, can help educators identify students who may be at risk of falling behind or dropping out. By analyzing various data points such as attendance, grades, and behavior patterns, predictive analytics models can flag students who may need additional support or intervention. This proactive approach allows educators to provide timely assistance to students in need, ultimately improving retention rates and student success.
Content Recommendation Systems
Machine learning algorithms are also used to develop content recommendation systems that suggest relevant learning materials, resources, and activities to students based on their preferences and performance. By personalizing the content delivery, these systems can enhance student engagement and motivation, making learning more interactive and enjoyable.
Conclusion
Machine learning applications in education have the potential to transform the way students learn and educators teach. By leveraging the power of machine learning algorithms, personalized learning experiences, adaptive learning platforms, intelligent tutoring systems, predictive analytics, and content recommendation systems, educational institutions can create more effective and engaging learning environments. As technology continues to advance, the integration of machine learning in education will play a crucial role in shaping the future of learning and unlocking the full potential of every student.