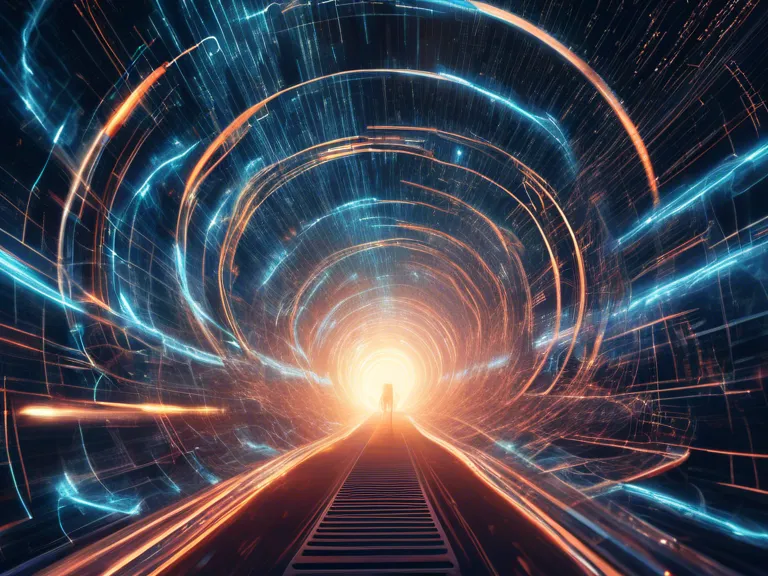
Introduction
Time travel has long been a fascinating concept in science fiction, allowing characters to journey backward or forward in time. But what if we could use machine learning to unravel temporal loops and predict events in the past or future? This intersection of machine learning and time travel opens up a realm of possibilities for research and exploration.
Understanding Temporal Loops
Temporal loops refer to situations where events in time become trapped in a repeating sequence, forming a loop. In the context of machine learning, temporal loops can present unique challenges and opportunities. By analyzing patterns and relationships in temporal data, machine learning algorithms can potentially identify and even exploit these loops for predictive purposes.
Machine Learning Algorithms for Time Travel
Several machine learning algorithms have been developed specifically for analyzing temporal data and predicting future events. Recurrent Neural Networks (RNNs) and Long Short-Term Memory (LSTM) networks are popular choices for capturing temporal dependencies in data sequences. These algorithms excel at processing time-series data and can potentially uncover patterns that lead to temporal loops.
Predictive Modeling in Time Travel
Using machine learning for time travel involves building predictive models that can forecast events based on historical data. By training algorithms on past data, these models can learn patterns and relationships that may indicate the existence of temporal loops. Through iterative training and testing, machine learning algorithms can improve their accuracy in predicting future events within the context of time travel.
Challenges and Considerations
While the idea of using machine learning for time travel is intriguing, it comes with several challenges and considerations. One of the main hurdles is the non-linear nature of time, which complicates the modeling and prediction process. Additionally, ethical concerns around altering the course of history or causing paradoxes must be carefully considered when delving into this research area.
Applications of Temporal Loops Machine Learning
The potential applications of temporal loops machine learning extend beyond science fiction. In fields such as finance, healthcare, and climate science, predictive modeling based on temporal data can offer valuable insights and improve decision-making. By leveraging machine learning techniques to unravel temporal loops, researchers can unlock new possibilities for understanding and shaping the future.
Conclusion
Unraveling temporal loops through machine learning in the context of time travel represents a cutting-edge intersection of technology and theoretical physics. While the concept may seem far-fetched, the advancements in machine learning and data analysis bring us closer to unlocking the mysteries of time. As researchers continue to explore this fascinating area of study, the possibilities for innovation and discovery are truly limitless.
askchrisknight.com
askchristopherknight.com
berkshirefoodjournal.com
cchirosw.com
coastalcottagesnl.com
destinationsite.com
finulldraft.com
gardenstatepaintingnj.com
impacthound.com
inneddi.com
itazurakoneko.com
judyforaz.com
localcarsnow.com
lovedayscinema.com
matiqistiqomahsambas.com
meetborofive.com
morskicovik.com
naturesrewardphotography.com
regenerativeresorts.com
restaurant-nashvilletn.com
scoopsicecreamtruck.com
simmonsmotorworks.com
trinityeco-tours.com
uncoverstudios.com
wong-multimedia.com
123exp-government.com
abozymes.com
atsheatingandair.com
bemjavim.com
caseintrend.com
cdnxbvn.com
charangolibre.com
denis-latin.com
frugal-science.com
fukuoka-kouyaren.com
hanabisou.com
herculessportcenter.com
highvoltageloja.com
hlwstoryboard.com
juderabig.com
leauxandbehold.com
melurix-next.com
moggiechicken.com
mytrendysins.com
nativeskinonline.com
ninethreestudio.com
pcmwereld.com
pichinkufibers.com
primerautobody.com
salmanhatta.com
shalevbrosh.com
sosorryrecords.com
sportsone-egy.com
wofome.com
ycadeau.com
abalawpc.com
airportpawnmiami.com
alchemycollaborative.com
blackfigdesigns.com
cubemedica.com
deliciousyarns.com
denledamtrancaocap.com
denledpanelcaocap.com
futebolrico.com
honsyuji.com
jayasupranaschool.com
jstact.com
kikichaos.com
knuckleupmartialarts.com
lavi-furniture.com
maisonsecomatiq.com
neighbors-construction.com
nspointers.com
opticiwear.com
richouli.com
soddydaisyhealthcare.com
soultalkmagazine.com
studywithpmouy.com
swordfightsandstarrynights.com
tamatur-bali.com
thepainreliefsecret.com
townhouselinens.com
vchainstore.com
vormaro.com
yukonlodgeforsale.com
airkoolny.com
an-geeke.com
bajka3.com
bertinoargenti.com
birolkitabevi.com
burikoland.com
emeraldseamless.com
floridanativecharters.com
iptvsportt.com
jupiterboutique.com
kateronline.com
kustomkoachrv.com
lademeuredufondateur.com
lucianamurta.com
luckydelaware.com
luckyindiana.com
lujannavas.com
mike4rep.com
milioshairsalon.com
movingrestaurantequipment.com
openseedvault.com
retrogradetours.com
revistaresiduos.com
roughandreadygrange.com
therusalka.com
toxnfill3.com
valleymaintenancelandscape.com
violetorlandi.com
wanpaku-golf.com
zinkdental.com
suryacctv.com
tuvannugioi.com
mandalynmusic.com
wireless-reviews.com
tussingblockwatch.com
greenteaphotography.com
jennierooney.com
vietamreview.net
kyavar.com
market-truth.com
alexandregaurier.com
omegagadget.com
banditosla.com
nursememama.com
oyunbilim.com
acme-nuclear.com
agilityimap.com
akikcombong.com
anniesmysteries.com
bflofoodie.com
brandzonestudios.com
cacemar.com
daperezlaw.com
denvyautomation.com
eugeneband.com
factory-eshop.com
florentdumas.com
hishaywireless.com
in2-signs.com
kuwekeza-holdings.com
mitaniya-ltd.com
mixfoure.com
mobilitypluspro2.com
moipravila.com
montreal-business-kit.com
mortiseandmiter.com
nextdigitaldental.com
nurdalilahputri.com
oem-phoneaccessories.com
palmbeachestepona.com
precavida.com
roscoeandetta.com
scriptsnmacros.com
sringserver.com
thecustomfairy.com
withlovefromangela.com
applebyandwood.com
auzigog.com
eac-w.com
homesbyelevation.com
nihilismforoptimists.com
slavonkandhortus.com
thekoreanpolitics.com
turningpointpt.com
val-up.com
wakansen.com
3dideation.com
achilles-fire.com
banatelhalal.com
biyografirehberi.com
bohams.com
comisiondeestudios.com
cooride-net.com
danayumul.com
ecadecom.com
edwardscornerfarmersmarket.com
ekspresif.com
ellajmae.com
ginroecooks.com
gracefueled.com
hightidekitchen.com
jeroenswolfs.com
marthalott.com
mollybroekman.com
mpthoidai.com
plumfashionpr.com
racktents.com
solzapower.com
southcoastbehavioralhealth.com
the101bali.com
thearguide.com
theartistfia.com
thefitnesswire.com
thelivelihoodproject.com
thelynndentonagency.com
wilkespoolsnspas.com
wjwatson.com
drinkganbei.com
mendenhallnews.com
nathaliemoliavko-visotzky.com
nationalinfertilityday.com
wide-aware.com
ashleymodernfurniture.com
babylonbusinessfinance.com
charliedewhirst.com
christianandmilitaryhats.com
hypnosisoneonone.com
icelandcomedyfilmfestival.com
kayelam.com
mlroadhouse.com
mumpreneursonline.com
posciesa.com
pursweets-and.com
rgparchive.com
therenegadehealthshow.com
travelingbitz.com
yutakaokada.com
22fps.com
aarondgraham.com
essentialaustin.com
femdotdot.com
harborcheese.com
innovar-env.com
mercicongo.com
oabphoto.com
pmptestprep.com
rmreflectivevest-jp.com
tempistico.com
filmintelligence.org
artisticbrit.com
avataracademyagency.com
blackteaworld.com
healthprosinrecovery.com
iancswanson.com
multiversecorpscomics.com
warrenindiana.com
growthremote.com
horizonbarcelona.com
iosdevcampcolorado.com
knoticalpr.com
kotaden.com
la-scuderia.com
nidoderatones.com
noexcuses5k.com
nolongerhome.com
oxfordcounselingcenter.com
phytacol.com
pizzaropizza.com
spotlightbd.com
tenbags.com
thetravellingwilbennetts.com
archwayintl.com
jyorganictea.com
newdadsplaybook.com
noahlemas.com
qatohost.com
redredphoto.com
rooms4nhs.com
seadragonenergy.com
spagzblox.com
toboer.com
aumantvmuseum.com
beyondausten.com
citylabstudio.com
diskonio.com
drinkcf.com
eft-dongle.com
emilymeganphotography.com
evolveathleticclub.com
godleystationvet.com
hirochanweb.com
homeonefurniture.com
ifiwasastylist.com
lacantinepopup.com
liriklagubatak.com
lo-ko.com
mensagenseatividades.com
myway-zeus.com
nevadadec.com
nokarikhabar.com
nuuuki.com
quenchpad.com
sckyrock.com
tindunghanoi.com
tradeshows-biz.com
wikimuzik.com